What is Pandas? A Guide to Data Analysis with Python
Pandas is one of the indispensable tools of data science and analysis, a powerful library that makes it easy to manipulate and analyze data with Python. If you are someone who wants to step into the world of data analysis, or if you are already working in this field and want to understand the Pandas library better; in both cases, you are in the right place! In this guide, we will cover in detail what Pandas is, how it works, its main features and how it can be used in data analysis processes.
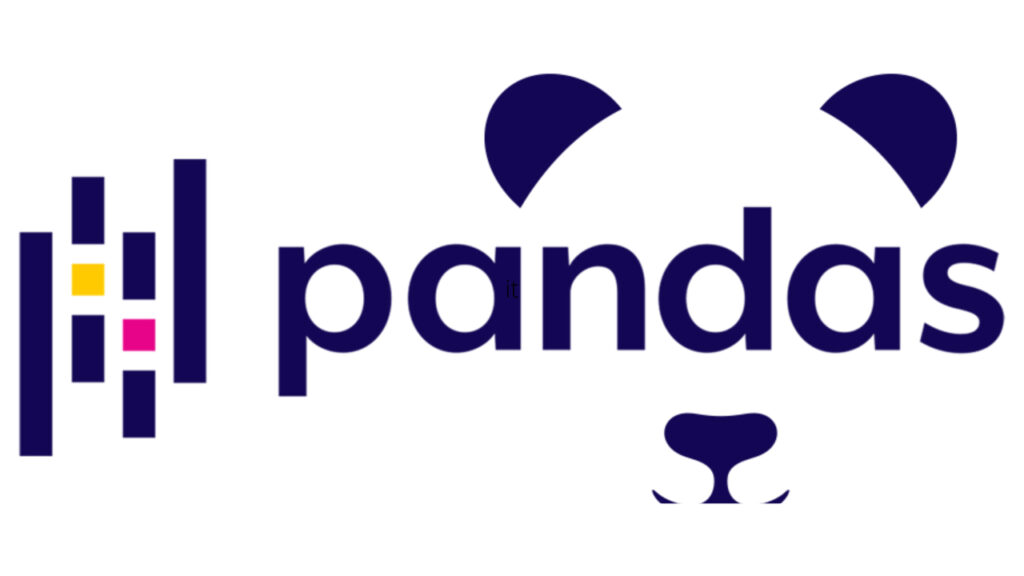
What is Pandas?
Pandas is an open source library for data analysis and manipulation in the Python programming language. Developed by Wes McKinney in 2008, Pandas is specifically designed to work with table-like data structures. It is frequently used by data scientists, financial analysts, data engineers and machine learning experts. Pandas is based on the NumPy library and enables working with large datasets in a fast, flexible and efficient way.
The biggest advantage of Pandas is that it facilitates data manipulation with data structures such as DataFrame and Series. These structures work in a similar way to Excel or SQL tables and allow you to quickly perform operations such as data cleaning, filtering, and grouping. In short, Pandas automates data analysis processes and allows you to solve complex data operations with a few lines of code.
Key Features
- DataFrame and Series: Organizes data in table and array format.
- Data Manipulation: Powerful tools for filtering, sorting, merging, etc.
- Missing Data Management: Easily identify and fill missing data.
- Data Visualization Integration: Compatibility with libraries such as Matplotlib and Seaborn.
- Fast Performance: Efficient operation even on large datasets.
Why Use It?
It provides flexible and powerful tools that can be used at every stage of data science. Here are the main areas where it is used:
- Data Cleansing: Real-world data is often messy. It may contain missing values, incorrect formats or inconsistencies. Pandas offers practical methods to solve such problems.
- Data Conversion: Ideal for transforming, grouping or summarizing data from one format to another.
- Data Analysis: Used for statistical analysis, trend analysis and correlation calculations.
- Data Visualization: Integrates with data visualization libraries, making it easy to turn your data into charts.
- Machine Learning: It is often preferred for data pre-processing in the process of preparing data for machine learning models.
For example, imagine you want to analyze sales data from an e-commerce company. With Pandas, you can upload this data, fill in missing values, examine sales trends and even visualize them. All this is done quickly thanks to its user-friendly structure.
Installation and Startup
Python must be installed before you start using Pandas. You can follow the steps below for installation:
Installation Steps
- Python Installation: Download and install the latest version of Python from python.org.
- Pandas Installation: Run the following command in terminal or command line:
pip install pandas
- NumPy Installation: Since Pandas depends on NumPy, it’s a good idea to install NumPy as well:
pip install numpy
Our First Code
Let’s do a simple example to test it. The following code creates a data frame and prints it to the screen:
import pandas as pd
# Sample data
data = {
"Name": ["Ali", "Ayşe", "Mehmet"],
"Age": [25, 30, 22],
"City": ["İstanbul", "Ankara", "İzmir"]
}
# Creating a DataFrame
df = pd.DataFrame(data)
# Printing a DataFrame
print(df)
Output:
Name Age City
0 Ali 25 İstanbul
1 Ayşe 30 Ankara
2 Mehmet 22 İzmir
This simple example shows how Pandas creates data frames and works in a table-like structure.
Basic Data Structures of Pandas: Series and DataFrame
Pandas has two basic data structures: Series and DataFrame. These structures are the cornerstones of data analysis processes.
Series
A Series is a one-dimensional data structure and is like a list or array. Each Series is an indexed column of data. For example
import pandas as pd
# Series creation
ages = pd.Series([25, 30, 22], index=["Ali", "Ayşe", "Mehmet"])
print(ages)
Output:
Ali 25
Ayşe 30
Mehmet 22
dtype: int64
Series represents a single column of data and works with indexes. This makes it easy to access data by name or number.
DataFrame
A DataFrame is a two-dimensional data structure and is like a table. It contains rows and columns. As we see in the example above, we can create a DataFrame by grouping multiple columns together. DataFrames work in a similar way to Excel or SQL tables and are ideal for complex data manipulations.
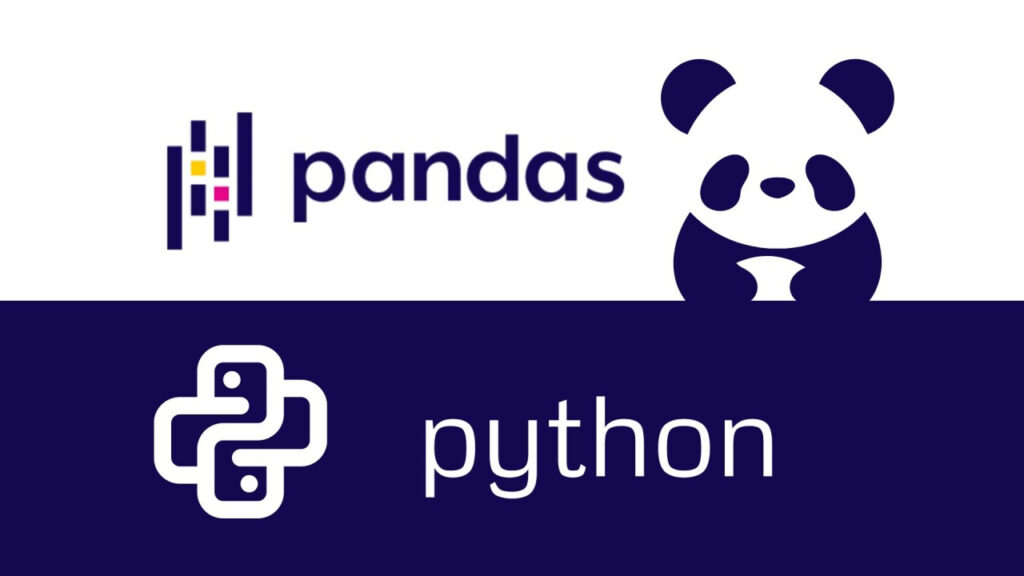
Data Manipulation with Pandas
It offers a wide set of tools for data manipulation. Here are the most common operations:
Data Loading
Pandas supports uploading data from different file formats: CSV, Excel, JSON, SQL, etc. For example, to upload a CSV file:
df = pd.read_csv("datas.csv")
print(df.head()) # Shows the first 5 lines
Data Filtering
To filter data according to certain conditions:
# Filter by age older than 25
filter = df[df["Age"] > 25]
print(filter)
Management of Missing Data
Missing data is a common problem in data analysis. Pandas provides methods to detect and manage missing data:
# Checking for missing data
print(df.isnull().sum())
# Filling missing data with averages
df["Age"].fillna(df["Age"].mean(), inplace=True)
Data Grouping and Summarization
To group data and extract summary statistics:
# Average age by city
average_age = df.groupby("City")["Age"].mean()
print(average_age)
Data Visualization
Pandas integrates with data visualization libraries (Matplotlib, Seaborn). For example, to create a bar chart:
import matplotlib.pyplot as plt
# Visualizing average age by city
average_age.plot(kind="bar", title="Average Age by City")
plt.show()
This code shows the average age by city as a bar chart. Pandas’ visualization tools help you quickly understand your data.
Advantages and Disadvantages
Advantages
- User-friendly and flexible API.
- High performance on large datasets.
- Compatibility with different data formats.
- Extensive documentation and broad community support.
Disadvantages
- Memory usage can be high in some cases.
- Performance optimization may be required for very large datasets.
- The learning curve can be a bit steep for beginners.
In conclusion, Pandas is one of the most powerful tools in the Python ecosystem for data analysis and manipulation. Data structures such as Series and DataFrame make life easier for data scientists with operations such as data loading, cleaning, filtering, and visualization. In this guide, we’ve covered Pandas’ key features, use cases and practical examples in detail. Whether you’re a data science enthusiast or a professional analyst, Pandas will give you superpowers when working with data!
If you want to continue learning Pandas, we encourage you to check out the official Pandas documentation or do projects with real-world data. If you have any questions, feel free to share them in the comments!